Lectures on the Nearest Neighbor Method for Data Sciences

The nearest neighbor method is a powerful technique for data classification and regression. It is a simple and intuitive method that can be used to solve a wide variety of problems. In this article, we will provide a comprehensive overview of the nearest neighbor method, covering its theoretical foundations, practical applications, and advantages and disadvantages. We will also discuss the different types of nearest neighbor algorithms and explore the challenges and future directions of research in this area.
The nearest neighbor method is based on the assumption that similar data points are likely to have similar outcomes. This assumption is known as the locality assumption. The nearest neighbor method works by finding the most similar data points to a new data point and then using the outcomes of these similar data points to predict the outcome of the new data point.
The similarity between data points can be measured using a variety of distance metrics. The most common distance metric is the Euclidean distance, which is the straight-line distance between two points. Other distance metrics include the Manhattan distance, which is the sum of the absolute differences between the coordinates of two points, and the cosine similarity, which is the cosine of the angle between two vectors.
4.2 out of 5
Language | : | English |
File size | : | 4563 KB |
Screen Reader | : | Supported |
Print length | : | 300 pages |
The number of nearest neighbors to use is a parameter that can be tuned to improve the performance of the nearest neighbor method. The optimal number of nearest neighbors depends on the data set and the problem being solved.
The nearest neighbor method has a wide range of practical applications in data sciences, including:
- Classification: The nearest neighbor method can be used to classify new data points into different categories. For example, the nearest neighbor method can be used to classify images of animals into different species.
- Regression: The nearest neighbor method can be used to predict the value of a continuous variable for a new data point. For example, the nearest neighbor method can be used to predict the price of a house based on the prices of similar houses in the same neighborhood.
- Clustering: The nearest neighbor method can be used to cluster data points into different groups. For example, the nearest neighbor method can be used to cluster customers into different segments based on their demographics and purchase history.
The nearest neighbor method has a number of advantages, including:
- Simplicity: The nearest neighbor method is a simple and intuitive method that is easy to implement.
- Versatility: The nearest neighbor method can be used to solve a wide variety of problems, including classification, regression, and clustering.
- Robustness: The nearest neighbor method is relatively robust to noise and outliers in the data.
However, the nearest neighbor method also has some disadvantages, including:
- Computational cost: The nearest neighbor method can be computationally expensive, especially for large data sets.
- Memory requirements: The nearest neighbor method requires storing the entire data set in memory, which can be a problem for large data sets.
- Sensitivity to the choice of distance metric and the number of nearest neighbors: The performance of the nearest neighbor method can be sensitive to the choice of distance metric and the number of nearest neighbors.
There are a number of different nearest neighbor algorithms, each with its own advantages and disadvantages. The most common nearest neighbor algorithms include:
- Brute-force search: The brute-force search algorithm is the simplest nearest neighbor algorithm. It works by iterating over all of the data points and finding the data point that is most similar to the new data point.
- KD-tree search: The KD-tree search algorithm is a more efficient nearest neighbor algorithm that uses a KD-tree to organize the data points. A KD-tree is a data structure that represents the data points as a tree, where each node in the tree represents a split in the data space.
- Ball tree search: The ball tree search algorithm is another efficient nearest neighbor algorithm that uses a ball tree to organize the data points. A ball tree is a data structure that represents the data points as a collection of balls, where each ball represents a region of the data space.
There are a number of challenges and future directions of research in the area of nearest neighbor methods. Some of the most important challenges include:
- Developing more efficient nearest neighbor algorithms: The nearest neighbor method can be computationally expensive, especially for large data sets. Developing more efficient nearest neighbor algorithms is an important area of research.
- Developing more robust nearest neighbor algorithms: The nearest neighbor method can be sensitive to noise and outliers in the data. Developing more robust nearest neighbor algorithms is another important area of research.
- Exploring new applications of nearest neighbor methods: The nearest neighbor method has a wide range of potential applications in data sciences. Exploring new applications of nearest neighbor methods is an important area of future research.
The nearest neighbor method is a powerful technique for data classification and regression. It is a simple and intuitive method that can be used to solve a wide variety of problems. However, the nearest neighbor method can also be computationally expensive and sensitive to the choice of distance metric and the number of nearest neighbors. There are a number of challenges and future directions of research in the area of nearest neighbor methods, including developing more efficient and robust algorithms and exploring new applications.
4.2 out of 5
Language | : | English |
File size | : | 4563 KB |
Screen Reader | : | Supported |
Print length | : | 300 pages |
Do you want to contribute by writing guest posts on this blog?
Please contact us and send us a resume of previous articles that you have written.
Novel
Chapter
Text
Genre
Paperback
E-book
Newspaper
Paragraph
Sentence
Bookmark
Shelf
Glossary
Preface
Synopsis
Footnote
Manuscript
Scroll
Bestseller
Classics
Narrative
Biography
Memoir
Dictionary
Thesaurus
Narrator
Character
Resolution
Borrowing
Periodicals
Study
Lending
Reading Room
Rare Books
Special Collections
Literacy
Study Group
Awards
Reading List
Theory
Textbooks
Gail Vaz Oxlade
Janie Noyes
Nancy Derey Riley
That Patchwork Place
Clover Autrey
Michelle Panayi
Catherine Hernandez
Mario Tonatiuh
Elizabeth Laban
Jason Weiss
Neil Fraser
Edmund M Burke
Sunil Kumar Kopparapu
James Hoag
C P Giuliani
Christopher Innes
Sandra Staines
Natasha Tremblay
Gary Giddins
Karen Fried
Light bulbAdvertise smarter! Our strategic ad space ensures maximum exposure. Reserve your spot today!
- Milton BellFollow ·4.1k
- Jack PowellFollow ·13k
- Osamu DazaiFollow ·19.5k
- Dominic SimmonsFollow ·17.2k
- Michael SimmonsFollow ·3.1k
- Aron CoxFollow ·19.5k
- Henry HayesFollow ·12.9k
- Jessie CoxFollow ·11.8k
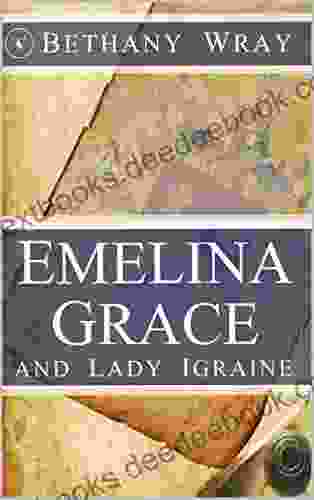

Unveiling the Enchanting Legends of Emelina Grace and...
Emelina Grace: The...
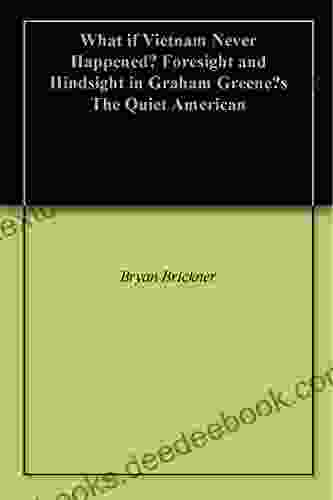

What If Vietnam Never Happened: Foresight and Hindsight...
Published in 1955, Graham Greene's The Quiet...
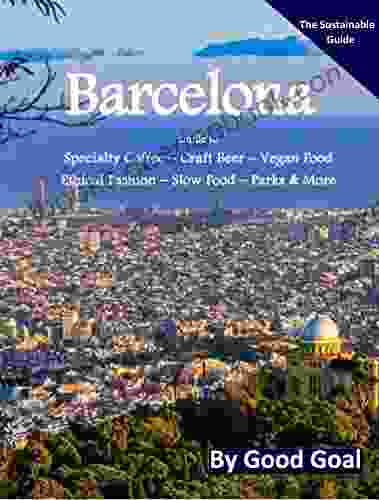

The Rise of Specialty Coffee, Craft Beer, Vegan Food,...
In recent years,...
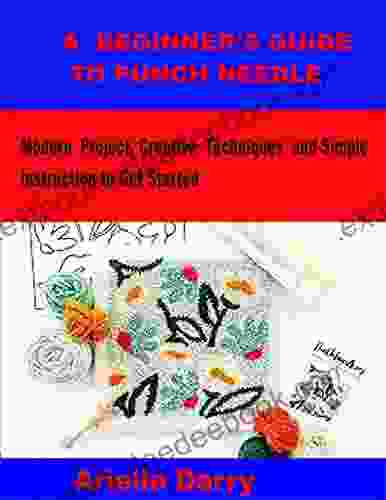

Modern Project Creative Techniques: A Comprehensive Guide...
In today's competitive business landscape,...
4.2 out of 5
Language | : | English |
File size | : | 4563 KB |
Screen Reader | : | Supported |
Print length | : | 300 pages |