Network Anomaly Detection: A Machine Learning Perspective

5 out of 5
Language | : | English |
File size | : | 13420 KB |
Print length | : | 366 pages |
Screen Reader | : | Supported |
In today's interconnected world, ensuring the security and integrity of our networks is paramount. Network anomalies, deviations from normal network behavior, can be indicative of malicious activities or system malfunctions, posing significant risks to organizations and individuals alike.
Network anomaly detection has emerged as a critical cornerstone of cybersecurity, enabling us to identify and respond to anomalies effectively and promptly. Traditional approaches to anomaly detection relied heavily on manual analysis and rule-based systems, which often proved inadequate in the face of increasingly sophisticated attacks and growing network complexity.
Machine learning (ML),with its ability to learn from vast amounts of data and recognize patterns, has revolutionized the field of network anomaly detection. By leveraging ML algorithms, we can automate the detection process, enhance accuracy, and adapt to evolving network environments.
Types of Machine Learning Algorithms for Network Anomaly Detection
- Supervised Learning:
- Unsupervised Learning:
Supervised learning algorithms are trained on labeled datasets, where each data point is associated with a known class or label. In the context of anomaly detection, the algorithm learns to identify normal network behavior based on labeled data and can then classify new, unlabeled data as either normal or anomalous.
Unsupervised learning algorithms, on the other hand, do not require labeled data. Instead, they uncover hidden patterns and structures within the data. Unsupervised learning is particularly useful in anomaly detection when labeled data is scarce or unavailable.
Challenges in Network Anomaly Detection
While ML offers significant benefits for network anomaly detection, it also presents several challenges:
- Big Data:
- Noise and Redundancy:
- Evolving Network Behavior:
- Performance Optimization:
Network traffic datasets can be extremely large, posing challenges for ML algorithms in terms of computational complexity and storage requirements.
Real-world network traffic often contains noise and redundancy, which can make it difficult to extract meaningful patterns and identify anomalies.
Network behavior is constantly evolving, making it crucial for ML algorithms to adapt to new patterns and behaviors over time.
ML algorithms must be optimized to balance accuracy, efficiency, and real-time processing requirements for effective anomaly detection.
Recent Advancements and Trends
Ongoing research and advancements in ML are driving new developments in network anomaly detection:
- Deep Learning:
- Graph-Based Anomaly Detection:
- Federated Learning:
Deep learning algorithms, with their ability to learn complex hierarchical representations of data, have shown promising results in anomaly detection.
Network traffic can be represented as a graph, where nodes represent hosts or devices and edges represent connections. Graph-based anomaly detection algorithms can exploit this structure to identify anomalous patterns and behaviors.
Federated learning enables ML models to be trained across multiple devices or networks without sharing sensitive data. This approach is particularly beneficial for anomaly detection in distributed or privacy-sensitive environments.
Network anomaly detection is essential for safeguarding networks from malicious activities and ensuring uninterrupted operation. Machine learning techniques offer powerful tools to automate the detection process, enhance accuracy, and adapt to evolving network environments. By leveraging ML algorithms, we can build robust and effective anomaly detection systems that protect our networks and data.
As the field of ML continues to advance, we can expect even more sophisticated and efficient network anomaly detection techniques to emerge, enabling us to stay ahead of evolving threats and maintain the integrity and security of our networks.
5 out of 5
Language | : | English |
File size | : | 13420 KB |
Print length | : | 366 pages |
Screen Reader | : | Supported |
Do you want to contribute by writing guest posts on this blog?
Please contact us and send us a resume of previous articles that you have written.
Novel
Page
Text
Genre
Library
E-book
Bibliography
Foreword
Preface
Footnote
Manuscript
Scroll
Codex
Bestseller
Classics
Biography
Autobiography
Memoir
Encyclopedia
Dictionary
Thesaurus
Narrator
Character
Resolution
Librarian
Catalog
Card Catalog
Borrowing
Stacks
Study
Research
Scholarly
Journals
Reading Room
Rare Books
Interlibrary
Thesis
Dissertation
Storytelling
Book Club
Jana Kirchner
Joseph Gutiz
Chris Enss
Denise Levertov
Sid Gustafson
Rita Monette
Ronald Reagan
Carl Hennicke
G On Tong
Robert J Mckee
Ruth Toor
Kent D Cleland
Joan Capafons
Eloisa James
Alastor Amalech
R Hal Williams
Richard H Robbins
Ludwig Thoma
Ali Noorani
1st Ed 2016 Edition Kindle Edition
Light bulbAdvertise smarter! Our strategic ad space ensures maximum exposure. Reserve your spot today!
- Oliver FosterFollow ·12.3k
- Brayden ReedFollow ·4.9k
- Finn CoxFollow ·13.6k
- Tom HayesFollow ·14.4k
- Camden MitchellFollow ·18.6k
- Sean TurnerFollow ·4.3k
- Tim ReedFollow ·19.4k
- Gavin MitchellFollow ·8.3k
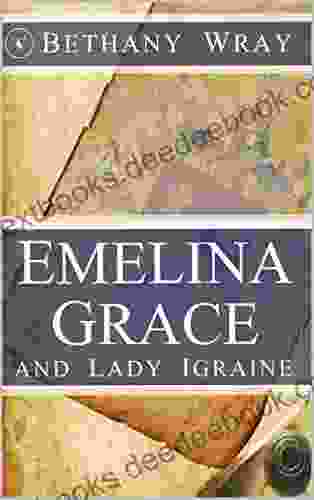

Unveiling the Enchanting Legends of Emelina Grace and...
Emelina Grace: The...
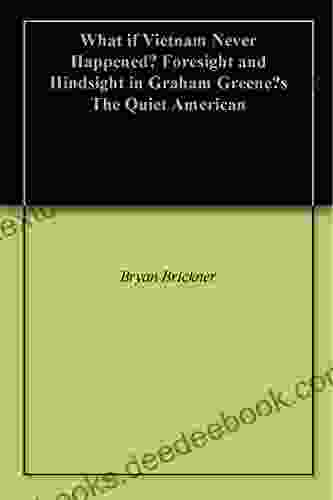

What If Vietnam Never Happened: Foresight and Hindsight...
Published in 1955, Graham Greene's The Quiet...
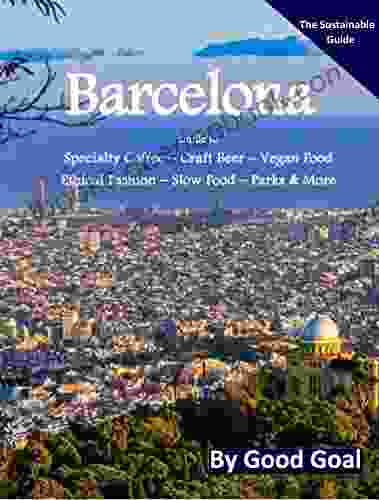

The Rise of Specialty Coffee, Craft Beer, Vegan Food,...
In recent years,...
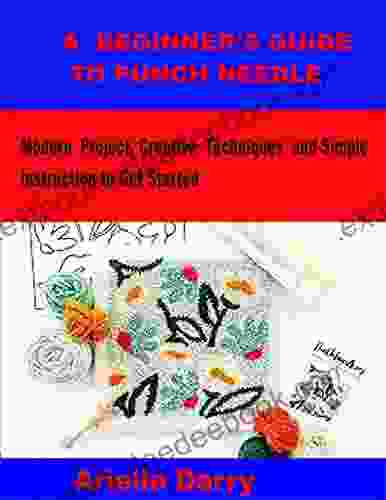

Modern Project Creative Techniques: A Comprehensive Guide...
In today's competitive business landscape,...
5 out of 5
Language | : | English |
File size | : | 13420 KB |
Print length | : | 366 pages |
Screen Reader | : | Supported |